Sponsor:
Air Force Office of Scientific Research (AFOSR), under the Department of Defense (DOD), under award number FA9550-12-1-0458; and National Institute of Standards and Technology (NIST), under Award No. 70NANB14H012.
Project Team Members:
Northwestern University
University of Michigan-Ann Arbor
Georgia Institute of Technology
Representation Design for Heterogeneous Microstructures
Overview
The goal of this project is to solve a key research question in designing microstructural materials systems: how to represent the microstructural design space quantitatively using a descriptor set that is sufficient yet small enough to be tractable.
In short, if all you have as the raw microstructure representation are the scanning electron microscopy (SEM) samples, as below --
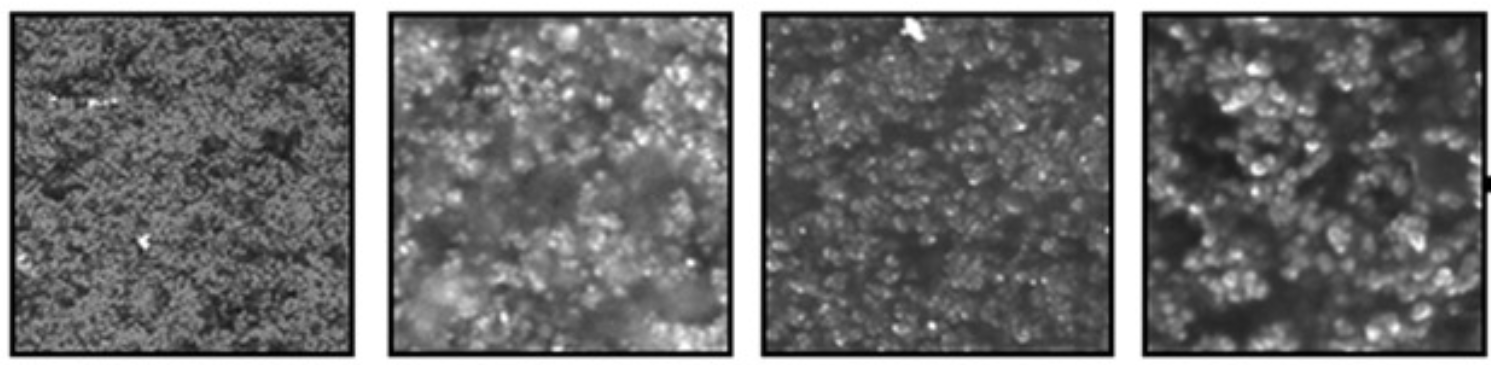
-- how to obtain a quantitative sense of how "good" this sample is, and subsequently, how to make it "better" in terms of certain property of interest.
Method
We develop a 4-step machine learning based method to exploit the microstructure-property database (Figure 2 below). The four steps include:
- Elimination of redundant descriptors using descriptor-descriptor correlation analysis;
- Microstructure correlation function-based supervised learning for further dimension reduction;
- Property-based supervised learning to identify key descriptors;
- Determination of microstructure design variables based on the optimization criteria of maximizing the impact score and minimizing the within-group correlations of the selected descriptor set.
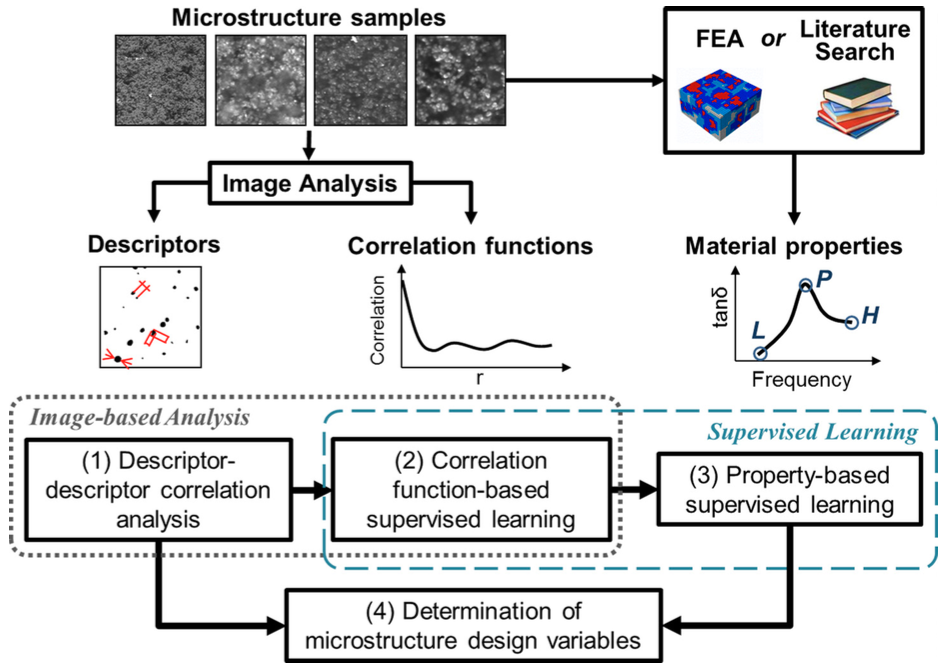
Steps 1 and 2 are image analysis-based procedures, which don't require expensive Finite Element Analysis (FEA) simulations. These two steps will provide a fast reduction of the size of a candidate descriptor set. Both steps 1 and 2 involve supervised learning. Step 3 needs structure-property data from either high-fidelity simulations or from literature. Step 4 is an optimization-based descriptor subset selection process.
Results
- In Step 1 we construct a correlation matrix to eliminate redundant descriptors, such as "surface area of filler phase" (highly correlated to "surface area of matrix phase"), and "average equivalent area" (highly correlated to "average equivalent radius").
- In Step 2 and Step 3 we further reduce the descriptor set using feature selection. Not only that, we show that using correlation function based feature ranking results in a highly similar outcome with property based. This again solidifies the belief that to obtaining knowledge about materials property can be done through observing the full representation of microstructure (e.g. through correlation functions).
- In Step 4, three microstructure design variables are determined by maximizing the descriptor set's impact score and minimizing the within-group correlation.
- We further validate the selected descriptors by performing an actual materials design with the three design variables. State-of-art computational design methods are applied to explore the microstructural design space to achieve optimal material properties.
Publications
- Hongyi Xu, Ruoqian Liu, Alok Choudhary, and Wei Chen. A Machine Learning-Based Design Representation Method for Designing Heterogeneous Microstructures. Journal of Mechanical Design, 137(5):051403–051403, ASME, May 2015. (pdf)
- Hongyi Xu, Ruoqian Liu, Alok Choudhary, and Wei Chen. A Machine Learning-Based Design Representation Method for Designing Heterogeneous Microstructures, (Design Automation (DAC) Best Paper). In the ASME International Design Engineering Technical Conferences, August 2014. (pdf)
Related Links
Acknowledgements
This work is supported by AFOSR (Air Force Office of Scientific Research), Department of Defense (DOD) under Award No. FA9550-12-1-0458; and by National Institute of Standards and Technology (NIST), under Award No. 70NANB14H012.